How Generative AI Helped Me Imagine a Better Robot
It didn’t give me schematics, but it did boost my creativity
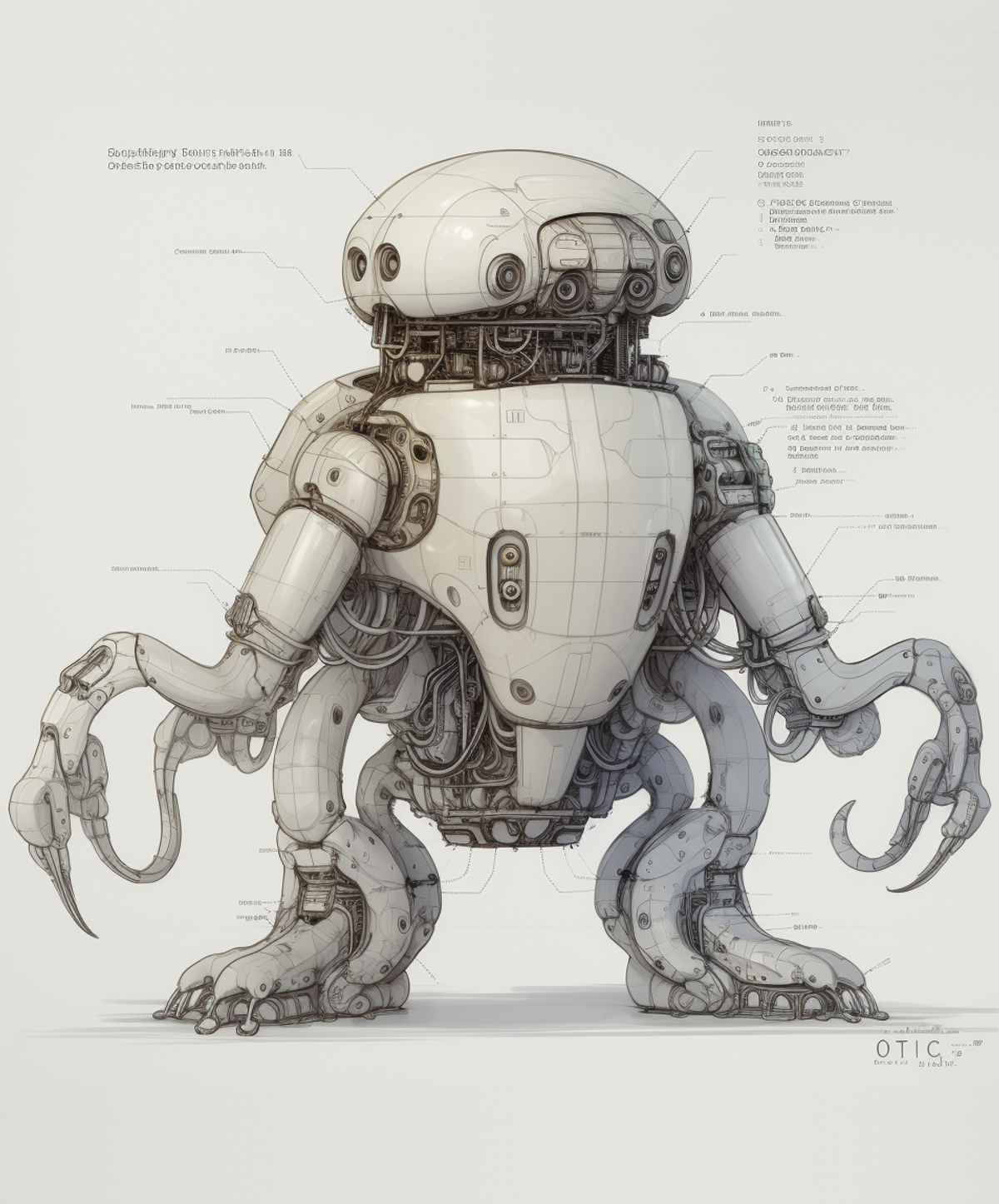
This year, 2023, will probably be remembered as the year of generative AI. It is still an open question whether generative AI will change our lives for the better. One thing is certain, though: New artificial-intelligence tools are being unveiled rapidly and will continue for some time to come. And engineers have much to gain from experimenting with them and incorporating them into their design process.
That’s already happening in certain spheres. For Aston Martin’s DBR22 concept car, designers relied on AI that’s integrated into Divergent Technologies’ digital 3D software to optimize the shape and layout of the rear subframe components. The rear subframe has an organic, skeletal look, enabled by the AI exploration of forms. The actual components were produced through additive manufacturing. Aston Martin says that this method substantially reduced the weight of the components while maintaining their rigidity. The company plans to use this same design and manufacturing process in upcoming low-volume vehicle models.
NASA research engineer Ryan McClelland calls these 3D-printed components, which he designed using commercial AI software, “evolved structures.” Henry Dennis/NASA
Other examples of AI-aided design can be found in NASA’s space hardware, including planetary instruments, space telescope, and the Mars Sample Return mission. NASA engineer Ryan McClelland says that the new AI-generated designs may “look somewhat alien and weird,” but they tolerate higher structural loads while weighing less than conventional components do. Also, they take a fraction of the time to design compared to traditional components. McClelland calls these new designs “evolved structures.” The phrase refers to how the AI software iterates through design mutations and converges on high-performing designs.
In these kinds of engineering environments, co-designing with generative AI, high-quality, structured data, and well-studied parameters can clearly lead to more creative and more effective new designs. I decided to give it a try.
How generative AI can inspire engineering design
Last January, I began experimenting with generative AI as part of my work on cyber-physical systems. Such systems cover a wide range of applications, including smart homes and autonomous vehicles. They rely on the integration of physical and computational components, usually with feedback loops between the components. To develop a cyber-physical system, designers and engineers must work collaboratively and think creatively. It’s a time-consuming process, and I wondered if AI generators could help expand the range of design options, enable more efficient iteration cycles, or facilitate collaboration across different disciplines.
Aston Martin used AI software to design parts for its DBR22 concept car. Aston Martin
When I began my experiments with generative AI, I wasn’t looking for nuts-and-bolts guidance on the design. Rather, I wanted inspiration. Initially, I tried text generators and music generators just for fun, but I eventually found image generators to be the best fit. An image generator is a type of machine-learning algorithm that can create images based on a set of input parameters, or prompts. I tested a number of platforms and worked to understand how to form good prompts (that is, the input text that generators use to produce images) with each platform. Among the platforms I tried were Craiyon, DALL-E 2, Midjourney, NightCafé, and Stable Diffusion. I found the combination of Midjourney and Stable Diffusion to be the best for my purposes.
Midjourney uses a proprietary machine-learning model, while Stable Diffusion makes its source code available for free. Midjourney can be used only with an Internet connection and offers different subscription plans. You can download and run Stable Diffusion on your computer and use it for free, or you can pay a nominal fee to use it online. I use Stable Diffusion on my local machine and have a subscription to Midjourney.
In my first experiment with generative AI, I used the image generators to co-design a self-reliant jellyfish robot. We plan to build such a robot in my lab at Uppsala University, in Sweden. Our group specializes in cyber-physical systems inspired by nature. We envision the jellyfish robots collecting microplastics from the ocean and acting as part of the marine ecosystem.
In our lab, we typically design cyber-physical systems through an iterative process that includes brainstorming, sketching, computer modeling, simulation, prototype building, and testing. We start by meeting as a team to come up with initial concepts based on the system’s intended purpose and constraints. Then we create rough sketches and basic CAD models to visualize different options. The most promising designs are simulated to analyze dynamics and refine the mechanics. We then build simplified prototypes for evaluation before constructing more polished versions. Extensive testing allows us to improve the system’s physical features and control system. The process is collaborative but relies heavily on the designers’ past experiences.
I wanted to see if using the AI image generators could open up possibilities we had yet to imagine. I started by trying various prompts, from vague one-sentence descriptions to long, detailed explanations. At the beginning, I didn’t know how to ask or even what to ask because I wasn’t familiar with the tool and its abilities. Understandably, those initial attempts were unsuccessful because the keywords I chose weren’t specific enough, and I didn’t give any information about the style, background, or detailed requirements.
In the author’s early attempts to generate an image of a jellyfish robot [image 1], she used this prompt:
underwater, self-reliant, mini robots, coral reef, ecosystem, hyper realistic.
The author got better results by refining her prompt. For image 2, she used the prompt:
jellyfish robot, plastic, white background.
Image 3 resulted from the prompt:
futuristic jellyfish robot, high detail, living under water, self-sufficient, fast, nature inspired.Didem Gürdür Broo/Midjourney
As the author added specific details to her prompts, she got images that aligned better with her vision of a jellyfish robot. Images 4, 5, and 6 all resulted from the prompt:
A futuristic electrical jellyfish robot designed to be self-sufficient and living under the sea, water or elastic glass-like material, shape shifter, technical design, perspective industrial design, copic style, cinematic high detail, ultra-detailed, moody grading, white background.Didem Gürdür Broo/Midjourney
As I tried more precise prompts, the designs started to look more in sync with my vision. I then played with different textures and materials, until I was happy with several of the designs.
It was exciting to see the results of my initial prompts in just a few minutes. But it took hours to make changes, reiterate the concepts, try new prompts, and combine the successful elements into a finished design.
Co-designing with AI was an illuminating experience. A prompt can cover many attributes, including the subject, medium, environment, color, and even mood. A good prompt, I learned, needed to be specific because I wanted the design to serve a particular purpose. On the other hand, I wanted to be surprised by the results. I discovered that I needed to strike a balance between what I knew and wanted, and what I didn’t know or couldn’t imagine but might want. I learned that anything that isn’t specified in the prompt might be randomly assigned to the image by the AI platform. And so if you want to be surprised about an attribute, then you can leave it unsaid. But if you want something specific to be included in the result, then you have to include it in the prompt, and you must be clear about any context or details that are important to you. You can also include instructions about the composition of the image, which helps a lot if you’re designing an engineering product.
It’s nearly impossible to control the outcome of generative AI
As part of my investigations, I tried to see how much I could control the co-creation process. Sometimes it worked, but most of the time it failed.
To generate an image of a humanoid robot [left], the author started with the simple prompt:
Humanoid robot, white background.
She then tried to incorporate cameras for eyes into the humanoid design [right], using this prompt:
Humanoid robot that has camera eyes, technical design, add text, full body perspective, strong arms, V-shaped body, cinematic high detail, light background.Didem Gürdür Broo/Midjourney
The text that appears on the humanoid robot design above isn’t actual words; it’s just letters and symbols that the image generator produced as part of the technical drawing aesthetic. When I prompted the AI for “technical design,” it frequently included this pseudo language, likely because the training data contained many examples of technical drawings and blueprints with similar-looking text. The letters are just visual elements that the algorithm associates with that style of technical illustration. So the AI is following patterns it recognized in the data, even though the text itself is nonsensical. This is an innocuous example of how these generators adopt quirks or biases from their training without any true understanding.
When I tried to change the jellyfish to an octopus, it failed miserably—which was surprising because, with apologies to any marine biologists reading this, to an engineer, a jellyfish and an octopus look quite similar. It’s a mystery why the generator produced good results for jellyfish but rigid, alien-like, and anatomically incorrect designs for octopuses. Again, I assume that this is related to the training datasets.
The author used this prompt to generate images of an octopus-like robot:
Futuristic electrical octopus robot, technical design, perspective industrial design, copic style, cinematic high detail, moody grading, white background.
The two bottom images were created several months after the top images and are slightly less crude looking but still do not resemble an octopus.
Didem Gürdür Broo/Midjourney
After producing several promising jellyfish robot designs using AI image generators, I reviewed them with my team to determine if any aspects could inform the development of real prototypes. We discussed which aesthetic and functional elements might translate well into physical models. For example, the curved, umbrella-shaped tops in many images could inspire material selection for the robot’s protective outer casing. The flowing tentacles could provide design cues for implementing the flexible manipulators that would interact with the marine environment. Seeing the different materials and compositions in the AI-generated images and the abstract, artistic style encouraged us toward more whimsical and creative thinking about the robot’s overall form and locomotion.
While we ultimately decided not to copy any of the designs directly, the organic shapes in the AI art sparked useful ideation and further research and exploration. That’s an important outcome because as any engineering designer knows, it’s tempting to start to implement things before you’ve done enough exploration. Even fanciful or impractical computer-generated concepts can benefit early-stage engineering design, by serving as rough prototypes, for instance. Tim Brown, CEO of the design firm IDEO, has noted that such prototypes “slow us down to speed us up. By taking the time to prototype our ideas, we avoid costly mistakes such as becoming too complex too early and sticking with a weak idea for too long.”
Even an unsuccessful result from generative AI can be instructive
On another occasion, I used image generators to try to illustrate the complexity of communication in a smart city.
Normally, I would start to create such diagrams on a whiteboard and then use drawing software, such as Microsoft Visio, Adobe Illustrator, or Adobe Photoshop, to re-create the drawing. I might look for existing libraries that contain sketches of the components I want to include—vehicles, buildings, traffic cameras, city infrastructure, sensors, databases. Then I would add arrows to show potential connections and data flows between these elements. For example, in a smart-city illustration, the arrows could show how traffic cameras send real-time data to the cloud and calculate parameters related to congestion before sending them to connected cars to optimize routing. Developing these diagrams requires carefully considering the different systems at play and the information that needs to be conveyed. It’s an intentional process focused on clear communication rather than one in which you can freely explore different visual styles.
The author tried using image generators to show complex information flow in a smart city, based on this prompt:
Figure that shows the complexity of communication between different components on a smart city, white background, clean design.Didem Gürdür Broo/Midjourney
I found that using an AI image generator provided more creative freedom than the drawing software does but didn’t accurately depict the complex interconnections in a smart city. The results above represent many of the individual elements effectively, but they are unsuccessful in showing information flow and interaction. The image generator was unable to understand the context or represent connections.
After using image generators for several months and pushing them to their limits, I concluded that they can be useful for exploration, inspiration, and producing rapid illustrations to share with my colleagues in brainstorming sessions. Even when the images themselves weren’t realistic or feasible designs, they prompted us to imagine new directions we might not have otherwise considered. Even the images that didn’t accurately convey information flows still served a useful purpose in driving productive brainstorming.
I also learned that the process of co-creating with generative AI requires some perseverance and dedication. While it is rewarding to obtain good results quickly, these tools become difficult to manage if you have a specific agenda and seek a specific outcome. But human users have little control over AI-generated iterations, and the results are unpredictable. Of course, you can continue to iterate in hopes that you’ll get a better result. But at present, it’s nearly impossible to control where the iterations will end up. I wouldn’t say that the co-creation process is purely led by humans—or not this human, at any rate.
I noticed how my own thinking, the way I communicate my ideas, and even my perspective on the results changed throughout the process. Many times, I began the design process with a particular feature in mind—for example, a specific background or material. After some iterations, I found myself instead choosing designs based on visual features and materials that I had not specified in my first prompts. In some instances, my specific prompts did not work; instead, I had to use parameters that increased the artistic freedom of the AI and decreased the importance of other specifications. So, the process not only allowed me to change the outcome of the design process, but it also allowed the AI to change the design and, perhaps, my thinking.
The image generators that I used have been updated many times since I began experimenting, and I’ve found that the newer versions have made the results more predictable. While predictability is a negative if your main purpose is to see unconventional design concepts, I can understand the need for more control when working with AI. I think in the future we will see tools that will perform quite predictably within well-defined constraints. More importantly, I expect to see image generators integrated with many engineering tools, and to see people using the data generated with these tools for training purposes.
Of course, the use of AI image generators raises serious ethical issues. They risk amplifying demographic and other biases in training data. Generated content can spread misinformation and violate privacy and intellectual property rights. There are many legitimate concerns about the impacts of AI generators on artists’ and writers’ livelihoods. Clearly, there is a need for transparency, oversight, and accountability regarding data sourcing, content generation, and downstream usage. I believe anyone who chooses to use generative AI must take such concerns seriously and use the generators ethically.
If we can ensure that generative AI is being used ethically, then I believe these tools have much to offer engineers. Co-creation with image generators can help us to explore the design of future systems. These tools can shift our mindsets and move us out of our comfort zones—it’s a way of creating a little bit of chaos before the rigors of engineering design impose order. By leveraging the power of AI, we engineers can start to think differently, see connections more clearly, consider future effects, and design innovative and sustainable solutions that can improve the lives of people around the world.
This article appears in the November 2023 print issue.